UPD Experts Determine the Optimal Model for Predicting Algal Blooms
Published: January 16, 2025
By: Harvey Sapigao
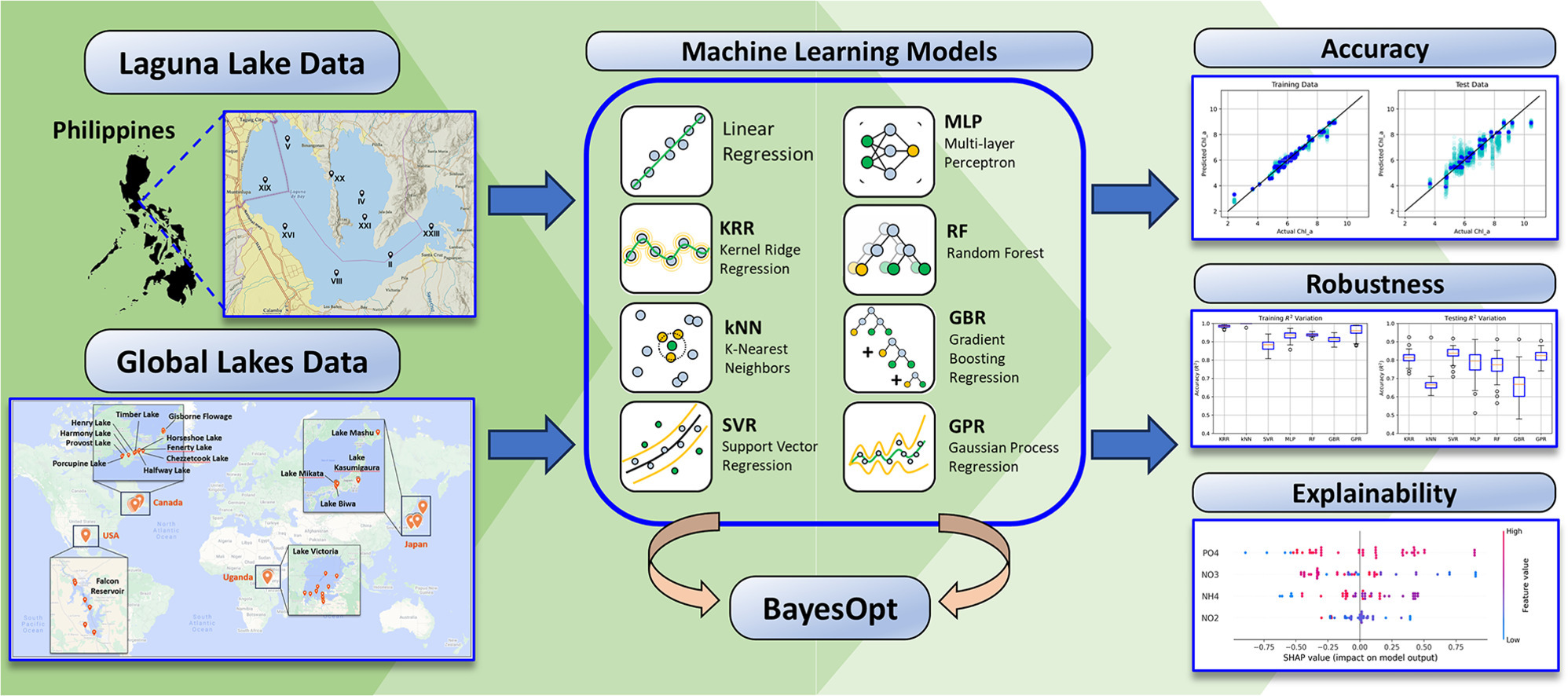
Algal blooms, which occur when algae overgrow in bodies of water, can not only turn the water green but also kill fish and contaminate the water supply of nearby communities. Laguna Lake, one of Metro Manila’s major sources of bangus and tilapia, as well as drinking water, is particularly prone to algal blooms, especially during El Niño.
A standard method for monitoring the algal population in water is to measure chlorophyll-a, the green pigment produced by algae. However, “If we wait for [the instruments] to indicate high algal content, it may already be too late since the bloom may have already occurred,” explains author Dr. Karl Ezra Pilario of the UPD Department of Chemical Engineering.
A more effective approach would be to monitor nitrate and phosphate concentrations in the water, as changes in these concentrations are often linked to increases in chlorophyll-a. Advanced tools such as machine learning (ML) models can be used to establish these complex relationships from data.
Since 1973, the Laguna Lake Development Authority (LLDA) has routinely monitored the lake’s water quality through remote sensing and monthly assessments. More recently, LLDA’s monitoring programs have employed mathematical tools and ML models. However, various models are currently available, and it is unclear which model suits Laguna Lake best.
Researchers from the University of the Philippines Diliman (UPD) recently published a study comparing the robustness and accuracy of eight common ML models for predicting algal blooms. Along with Dr. Pilario, Dr. Maria Pythias Espino of the UP Diliman College of Science Institute of Chemistry (UPD-CS IC), and Dr. Aurelio de los Reyes V and Eric Jan Escober of the UPD-CS Institute of Mathematics (IM) used water quality data from Laguna Lake and historical data from global lakes to train these models.
Of the eight, they discovered that two models called the Kernel Ridge Regression (KRR) and Gaussian Process Regression (GPR), performed better than others. These models both belong to a class called similarity-based models, which “use the philosophy that similar-looking inputs must give similar-looking outputs,” explained Dr. Pilario.
The other models included tree-based models, which function like a decision-making flowchart, and artificial neural nets, a framework inspired by our brains’ neural networks.
Although all models achieved high accuracy, KRR was the most accurate for Laguna Lake, while GPR was the best for global lakes. Moreover, KRR and GPR were more robust than the other models, allowing them to handle noisy data more effectively.
“Now that we have an accurate, robust, and explainable predictor of chlorophyll-a, we can deploy the model for rapid detection of impending algal blooms,” said Dr. Pilario. “We can take a water sample from the lake at any time, bring it to the lab to obtain the current nitrate ion and phosphate ion content, then estimate the chlorophyll-a from these values using KRR or GPR.”
“We recommend monthly monitoring of these values so that if an impending algal bloom is detected, there is ample time to prepare for interventions or mitigation strategies,” added Dr. Pilario.
While KRR and GPR can now be used for algal bloom prediction, the researchers noted that there are still many ways to improve the models. For instance, they are considering additional predictors like weather conditions, land cover types, and other effects caused by humans. Since samples from Laguna Lake were collected in just one season, they also plan to test the models with samples taken at different times of the year.
“Lastly, on the modeling side, we need to test more models that might be more accurate than KRR or GPR,” concluded Dr. Pilario. “In the future, we encourage researchers to test for the robustness and explainability of their machine learning models, and not just for accuracy, because it helps make the results more believable for policy-making.”
For interview requests and other concerns, please contact media@science.upd.edu.ph
References:
Pilario, K. E., Escober, E. J., De Los Reyes, A., V., & Espino, M. P. (2024). Robust Prediction of Chlorophyll-a from Nitrogen and Phosphorus Content in Philippine and Global Lakes Using Fine-Tuned, Explainable Machine Learning. Environmental Challenges, 101056. https://doi.org/10.1016/j.envc.2024.101056